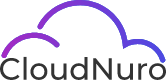
Book a Demo
Thank you! Your submission has been received!
Oops! Something went wrong while submitting the form.
Introduction: The Rise of AI Risk Governance
Generative AI (GenAI) has shifted from a technological novelty to a foundational capability for enterprises. In 2024 alone, surveys by Gartner and IDC indicate that over 60% of enterprises incorporated GenAI into at least one business-critical process. From intelligent chatbots and AI-powered decision-support systems to code generation and content creation, GenAI is everywhere.
However, this adoption has not come without consequences. Recent cases, such as major financial institutions fined for unauthorized AI model usage, or data leakage incidents involving customer support chatbots, illustrate the expanding risk landscape. Organizations face legal, operational, and reputational threats as GenAI models are inherently non-deterministic, can hallucinate, propagate bias, or expose sensitive data.
Compounding this challenge, regulators worldwide are acting fast. The EU AI Act (enforceable from mid-2025) introduces strict governance, especially for high-risk and general-purpose AI models. The NIST AI Risk Management Framework (AI RMF) and ISO/IEC 42001 further standardize AI risk management practices, making AI risk governance unavoidable.
Yet, most organizations today still rely on traditional GRC (Governance, Risk, Compliance) and security tools that lack AI-native capabilities. These platforms typically cannot:
This gap is why AI Risk Governance Platforms have emerged as essential. These tools enable CISOs, AI Governance Leads, Risk Managers, and Compliance Officers to manage the AI lifecycle — from model onboarding to continuous monitoring — ensuring responsible, secure, and compliant AI usage.
What is AI Risk Governance?
AI Risk Governance refers to the structured set of practices, processes, and tools used to identify, assess, mitigate, and monitor AI-related risks. It is especially critical for Generative AI, which introduces unique challenges, including:
Key Components of AI Risk Governance
Why Traditional GRC Is Not Enough
Traditional GRC tools focus on policies, risk registers, and audits. However, AI governance requires:
Must-Have Features in AI Risk Governance Tools
1. AI Model Inventory & Classification
Automatically discover AI models used internally and externally. Categorize them by type (Foundation Models, GenAI, Predictive Models), data sensitivity, and risk tier.
2. Generative AI Usage Monitoring
Monitor real-time usage of GenAI APIs, LLM-based tools (OpenAI, Anthropic, Google Gemini), and AI agents across SaaS platforms, cloud environments, and internal apps.
3. Regulatory Framework Mapping
Built-in alignment with the EU AI Act (including Annex III risk categories), NIST AI RMF functions (Govern, Map, Measure, Manage), and ISO/IEC 42001 requirements.
4. Automated AI Risk Assessments
Perform model-specific risk assessments for bias, explainability, fairness, robustness, privacy, and security.
5. Control & Policy Enforcement
Implement usage controls:
6. Audit-Ready Documentation
Generate and maintain documentation that meets internal audit, regulatory, and third-party assurance needs.
7. Seamless Integration
Native integrations with IAM (Okta, Azure AD), GRC (ServiceNow, Archer), SIEM, CSPM, and DevSecOps pipelines.
Top 10 AI Risk Governance Tools (2025 Guide)
1. IBM watsonx.governance
IBM watsonx.governance is an enterprise-grade AI governance solution designed to help organizations manage AI risks across their AI and ML lifecycle. Built on IBM's watsonx platform, it provides AI-specific governance covering explainability, bias detection, risk scoring, and robust regulatory alignment (EU AI Act, NIST AI RMF). The tool is designed to support organizations already invested in the IBM and Red Hat ecosystems, offering seamless integration with IBM’s AI lifecycle tooling.\n\nKey Modules
Integrations
Pros
Cons
Ideal Use-Case
Large enterprises needing end-to-end AI lifecycle and risk management with pre-built integration into IBM’s AI stack.
Screenshot:
2. Credo AI
Credo AI focuses on Responsible AI and regulatory compliance. Unlike traditional AI monitoring platforms, Credo AI enables both technical and non-technical teams to manage AI risks collaboratively. Its governance engine helps organizations perform structured AI risk assessments, enforce AI policies, and align with frameworks like the EU AI Act and NIST AI RMF. Credo AI is also known for strong support in generating audit-ready documentation and impact assessments.\n\nKey Modules
Integrations
Pros
Cons
Ideal Use-Case
Mid-market and enterprise organizations prioritizing responsible AI governance and regulatory compliance preparation.
Screenshot:
3. CalypsoAI
CalypsoAI is purpose-built for real-time GenAI usage monitoring, prompt inspection, and policy enforcement. It focuses on securing LLM-based applications by offering runtime risk management, including logging, alerting, and blocking of unsafe or unauthorized GenAI usage. It is highly favored by security teams integrating AI risk into their SOC operations.\n\nKey Modules
Integrations
Pros
Cons
Ideal Use-Case
Security, Risk, and Compliance teams requiring operational control of GenAI usage across cloud, SaaS, and internal environments.
Screenshot:
4. Truera
Truera is an AI Quality and Explainability platform focused on improving model transparency and fairness. Unlike many AI governance tools that focus solely on documentation and compliance, Truera provides direct insights into model behavior, explainability, fairness, and bias detection. It is often used alongside AI governance platforms to strengthen model-level insights and diagnostics, especially for high-stakes AI applications.
Key Modules
Integrations
Pros
Cons
Ideal Use-Case
Organizations building or consuming high-stakes AI models (credit scoring, healthcare, HR) that require explainability and fairness assurance.
Screenshot:
5. Holistic AI
Holistic AI is purpose-built for AI governance and regulatory alignment. It is one of the first platforms to integrate AI-specific policy enforcement with automated compliance checks aligned with the EU AI Act and NIST AI RMF. It offers risk assessments, documentation automation, and regulatory mapping for high-risk AI systems.
Key Modules
Integrations
Pros
Cons
Ideal Use-Case
Enterprises preparing for EU AI Act or ISO/IEC 42001 compliance with multiple AI use-cases across business units.
Screenshot:
6. Bain FRIDA
Bain & Company’s FRIDA (Framework for Responsible, Inclusive, and Ethical AI) is not a software tool but a full AI governance framework and methodology delivered via Bain's consulting engagements. FRIDA helps enterprises design AI governance programs, conduct AI risk assessments, and establish AI operating models aligned with emerging regulations.
Key Modules
Integrations
Pros
Cons
Ideal Use-Case
Enterprises needing to design or revamp their AI governance and Responsible AI programs from scratch.
Screenshot:
7. Fiddler AI
Fiddler AI provides a robust AI Observability Platform offering model monitoring, explainability, and risk detection. It is focused on AI reliability and performance rather than regulatory compliance alone. Fiddler is widely used by data science and AI engineering teams to ensure AI model robustness and fairness in production.
Key Modules
Integrations
Pros
Cons
Ideal Use-Case
AI engineering and data science teams focusing on improving model performance, explainability, and trust.
Screenshot:
8. Aporia
Aporia is a lightweight AI monitoring and observability platform designed for fast deployment and ease of use. It offers real-time monitoring, fairness checks, and anomaly detection with a focus on simplicity and developer-friendly workflows. While less comprehensive on the governance side, it provides essential monitoring capabilities for fast-moving AI teams.
Key Modules
Integrations
Pros
Cons
Ideal Use-Case
Startups and AI teams seeking rapid monitoring and alerting without full AI governance overhead.
Screenshot:
9. Monitaur
Monitaur is a governance platform tailored specifically for financial services, insurance, and healthcare sectors. It focuses on auditability, documentation, and model risk management, enabling highly regulated organizations to meet internal and external audit requirements. Monitaur is often used as an “AI Audit System of Record.”
Key Modules
Integrations
Pros
Cons
Ideal Use-Case
Highly regulated industries requiring audit-ready AI risk management.
Screenshot:
10. Tonic.ai
Tonic.ai is a synthetic data generation platform focused on privacy-preserving AI development. It helps organizations mitigate data privacy risks by generating high-quality synthetic datasets for AI model training and testing. While not a full AI governance suite, it is a valuable tool for AI privacy risk mitigation.
Key Modules
Integrations
Pros
Cons
Ideal Use-Case
Organizations focused on privacy-enhancing AI development, especially in healthcare, finance, or regulated data environments.
Screenshot:
Comparison Table:
Best Practices for AI Risk Governance
1. Establish a Formal AI Governance Structure
Effective AI governance starts with dedicated governance structures. Leading organizations establish AI Risk Committees comprising cross-functional members from Security, Risk, Compliance, AI/ML teams, Legal, and Business Units. This committee should own the AI risk policy, continuously monitor AI use cases, and make decisions on acceptable use, risk thresholds, and third-party AI vendor assessments.
2. Integrate AI Governance into Existing IT & Security Frameworks
AI governance should not exist as an isolated function. Mature organizations align AI governance with their existing GRC (Governance, Risk, Compliance), IAM (Identity & Access Management), Data Governance, and Cloud Security programs. AI controls should be extensions of existing access controls, audit logs, incident response workflows, and compliance reporting.
For example, GenAI usage detected via API logs could be integrated into SIEM systems, allowing security teams to detect anomalies and enforce controls.
3. Prioritize Continuous Monitoring and Dynamic Risk Assessment
Static risk assessments at the time of AI model deployment are insufficient. AI usage patterns change, models evolve, and new threats emerge. AI Risk Governance platforms must continuously monitor:
Implement automated risk scoring and re-assessment cycles aligned with significant changes in AI pipelines.
4. Engage Legal, Compliance, and Privacy Teams Early
Legal and privacy risks in AI (e.g., GDPR non-compliance, intellectual property exposure) are as critical as technical risks. Best practice is to integrate legal and compliance reviews before models are approved for production and whenever AI-powered products are updated.
5. Define Prompt Management and Usage Policies
Organizations must go beyond generic acceptable-use policies and create:
6. Link AI Governance to Regulatory Obligations
Successful organizations proactively map AI usage and governance practices to the EU AI Act, NIST AI RMF, and ISO/IEC 42001. They use platforms that maintain up-to-date regulatory mappings and evidence collection to reduce audit preparation overhead.
Common Pitfalls
1. Blind Spots in AI Usage
Many organizations suffer from “shadow AI,” where employees use GenAI tools (e.g., ChatGPT, Midjourney) without formal approval. These tools may process sensitive data without the organization’s knowledge. Without GenAI usage monitoring integrated into cloud and network controls, enterprises face significant data leakage risks.
2. Over-Reliance on Traditional GRC and Security Tools
CISOs often assume their GRC, SIEM, and IAM tools will suffice for AI governance. However, these platforms were not designed to:
This results in critical blind spots.
3. Treating AI Governance as a One-Time Exercise
AI Risk Governance is not a set-it-and-forget-it process. AI models may be retrained, integrated into new applications, or exposed to evolving threats. Without continuous risk assessments and governance updates, AI risks accumulate silently.
4. Poor Integration with Enterprise Security
Even the most robust AI governance policies fail if they do not integrate with existing identity, access, and monitoring systems. AI usage controls must tie into IAM policies, cloud-native security services, and incident response workflows to be enforceable.
5. Underestimating the Complexity of Regulatory Compliance
Many organizations underestimate the depth of the EU AI Act, NIST AI RMF, and ISO/IEC 42001 requirements. It is not enough to state “AI is governed”; enterprises must:
Neglecting these requirements can result in fines, legal risks, and reputational damage.
Choosing the Right Tool
Selecting an AI Risk Governance solution is not a one-size-fits-all exercise. Enterprises should consider:
Conclusion
2025 is the tipping point for AI risk governance. With the EU AI Act, NIST AI RMF, and ISO/IEC 42001 shaping global compliance expectations, organizations cannot afford to treat AI risk as an afterthought. Generative AI brings transformative power—but also complex, rapidly evolving risks.
Enterprises need more than just AI governance; they need AI + SaaS + Cloud Governance working hand in hand.
This is where CloudNuro becomes a strategic partner.
CloudNuro provides visibility into SaaS and Cloud environments, enabling you to:
Whether you are building your AI governance program or looking to strengthen it with SaaS and Cloud insights, CloudNuro helps you close the gaps that traditional tools miss.
Ready to operationalize AI Governance and SaaS Governance together?
Book a demo with our team to see how CloudNuro can help you:
👉 Schedule Your Demo Today
Request a no cost, no obligation free assessment —just 15 minutes to savings!
Get StartedRecognized Leader in SaaS Management Platforms by Info-Tech SoftwareReviews